Drug Toxicity Prediction Using Integrated Graph Neural Networks and Attention-Based Random Walk Algorithm
Vol. 52, No. 3, pp. 234-240, Mar. 2025

-
drug toxicity prediction Graph Neural Network Attention Mechanisms random walk Artificial intelligence
Abstract
Statistics
Cumulative Counts from November, 2022
Multiple requests among the same browser session are counted as one view. If you mouse over a chart, the values of data points will be shown.
Multiple requests among the same browser session are counted as one view. If you mouse over a chart, the values of data points will be shown.
|
Cite this article
[IEEE Style]
J. Park, J. Chu, Y. Cho, "Drug Toxicity Prediction Using Integrated Graph Neural Networks and Attention-Based Random Walk Algorithm," Journal of KIISE, JOK, vol. 52, no. 3, pp. 234-240, 2025. DOI: 10.5626/JOK.2025.52.3.234.
[ACM Style]
Jong-Hoon Park, Jae-Woo Chu, and Young-Rae Cho. 2025. Drug Toxicity Prediction Using Integrated Graph Neural Networks and Attention-Based Random Walk Algorithm. Journal of KIISE, JOK, 52, 3, (2025), 234-240. DOI: 10.5626/JOK.2025.52.3.234.
[KCI Style]
박종훈, 추재우, 조영래, "Graph Neural Network 통합 및 어텐션 기반 랜덤워크 알고리즘을 이용한 약물 독성 예측," 한국정보과학회 논문지, 제52권, 제3호, 234~240쪽, 2025. DOI: 10.5626/JOK.2025.52.3.234.
[Endnote/Zotero/Mendeley (RIS)] Download
[BibTeX] Download
Search
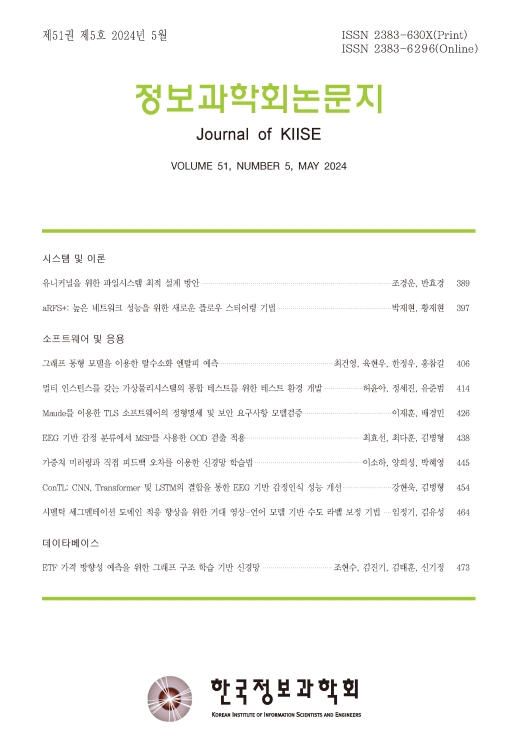
Journal of KIISE
- ISSN : 2383-630X(Print)
- ISSN : 2383-6296(Electronic)
- KCI Accredited Journal
Editorial Office
- Tel. +82-2-588-9240
- Fax. +82-2-521-1352
- E-mail. chwoo@kiise.or.kr