Motor Imagery EEG Classification Method using EMD and FFT
Vol. 41, No. 12, pp. 1050-1057, Dec. 2014
-
brain-computer interface Electroencephalogram motor imagery empirical mode decomposition Fast Fourier transform
Abstract
Statistics
Cumulative Counts from November, 2022
Multiple requests among the same browser session are counted as one view. If you mouse over a chart, the values of data points will be shown.
Multiple requests among the same browser session are counted as one view. If you mouse over a chart, the values of data points will be shown.
|
Cite this article
[IEEE Style]
D. Lee, H. Lee, S. Lee, "Motor Imagery EEG Classification Method using EMD and FFT," Journal of KIISE, JOK, vol. 41, no. 12, pp. 1050-1057, 2014. DOI: .
[ACM Style]
David Lee, Hee-Jae Lee, and Sang-Goog Lee. 2014. Motor Imagery EEG Classification Method using EMD and FFT. Journal of KIISE, JOK, 41, 12, (2014), 1050-1057. DOI: .
[KCI Style]
이다빛, 이희재, 이상국, "EMD와 FFT를 이용한 동작 상상 EEG 분류 기법," 한국정보과학회 논문지, 제41권, 제12호, 1050~1057쪽, 2014. DOI: .
[Endnote/Zotero/Mendeley (RIS)] Download
[BibTeX] Download
Search
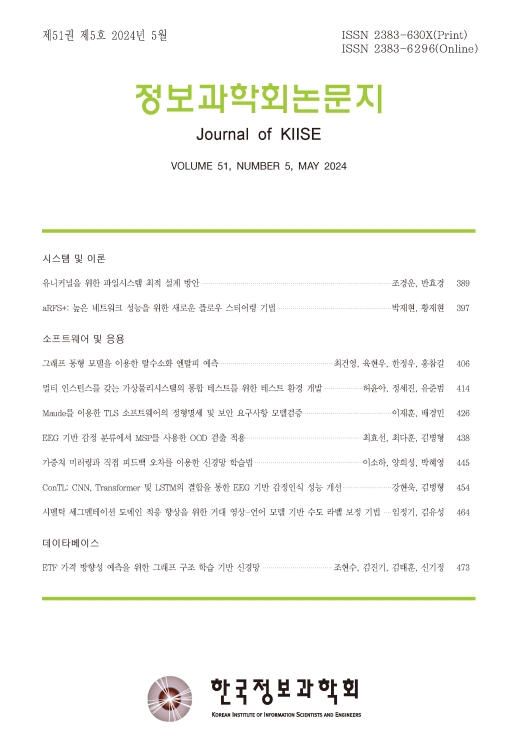
Journal of KIISE
- ISSN : 2383-630X(Print)
- ISSN : 2383-6296(Electronic)
- KCI Accredited Journal
Editorial Office
- Tel. +82-2-588-9240
- Fax. +82-2-521-1352
- E-mail. chwoo@kiise.or.kr