Digital Library[ Search Result ]
The Multivariate Sensor Data Classification using Time Series Imaging
http://doi.org/10.5626/JOK.2022.49.8.593
Various methods have been proposed in order to predict the future, from statistical-based time series analysis methods to deep learning-based prediction models, such as LSTM. However, the real industry data are highly complex due to various unpredictable factors. Therefore, it is difficult for the prediction models alone to extract valuable information from the data. Time series imaging is a method for converting time series into two-dimensional images, enabling the extraction of information that is difficult to interpret from raw data. In this paper, we transform the multivariate sensor data into two-dimensional multichannel images, and based on them, we propose a time series classification method. Furthermore, we compare the proposed method with the previous time series prediction methods to verify its usefulness.
Double-Averaging Acceleration with Synchronization Barrier Repositioning and Pipelining in Deep Learning
http://doi.org/10.5626/JOK.2021.48.11.1221
In deep learning using distributed computing, synchronization is one of the most important factors. While Local SGD is a low-frequency synchronization method that enables fast training, it is limited by high convergence difficulties. And Double-Averaging and SlowMo have been proposed to reduce the convergence difficulties of Local SGD. Double-Averaging improves the convergence difficulties by adding momentum buffer synchronization. However, the training time also increases due to the increased data synchronization. On the other hand, SlowMo adds a Two-layer momentum structure to the Local SGD resulting in reduced convergence difficulties without additional synchronization. However, this requires finding the appropriate SlowMo hyper-parameters. Therefore, in this paper, we proposed accelerated Double-Averaging via synchronization barrier repositioning and pipelining. The proposed method significantly reduced the convergence difficulties and accelerated performance.
Search
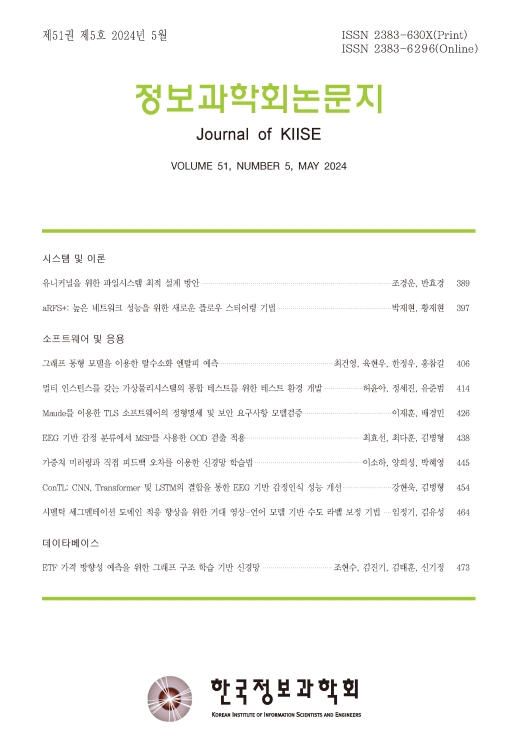
Journal of KIISE
- ISSN : 2383-630X(Print)
- ISSN : 2383-6296(Electronic)
- KCI Accredited Journal
Editorial Office
- Tel. +82-2-588-9240
- Fax. +82-2-521-1352
- E-mail. chwoo@kiise.or.kr