Digital Library[ Search Result ]
An Automatic Framework for Nested Normalization and Table Migration of Large-Scale Hierarchical Data
Dasol Kim, Myeong-Seon Gil, Heesun Won, Yang-Sae Moon
http://doi.org/10.5626/JOK.2023.50.6.521
In the open data portal, a lot of data is distributed in the hierarchical structure of JSON and XML formats, and the scale is very large. Such hierarchical data includes several nestings because of its structural characteristics. As a result, nested table normalization and scale limitation problems can occur, which limits the utilization of large-scale open data. In this paper, we adopt Airbyte, an open-source ELT platform, for table migration of hierarchical files, and propose a new framework for automating table migration. This is the first study to report Airbyte’s nested JSON handling issue and contribute to solving the issue. Through extensive evaluation of the proposed framework for actual US data portals, we show that it operates normally even for structures that include multiple nestings, and it can process large-scale migration of 1.6K or more by providing automated processing logic. These results mean that the proposed framework is a very practical one that supports the nested normalization of hierarchical data and provides a reliable large-scale migration function.
Secure Multiparty Computation of Principal Component Analysis
Sang-Pil Kim, Sanghun Lee, Myeong-Seon Gil, Yang-Sae Moon, Hee-Sun Won
In recent years, many research efforts have been made on privacy-preserving data mining (PPDM) in data of large volume. In this paper, we propose a PPDM solution based on principal component analysis (PCA), which can be widely used in computing correlation among sensitive data sets. The general method of computing PCA is to collect all the data spread in multiple nodes into a single node before starting the PCA computation; however, this approach discloses sensitive data of individual nodes, involves a large amount of computation, and incurs large communication overheads. To solve the problem, in this paper, we present an efficient method that securely computes PCA without the need to collect all the data. The proposed method shares only limited information among individual nodes, but obtains the same result as that of the original PCA. In addition, we present a dimensionality reduction technique for the proposed method and use it to improve the performance of secure similar document detection. Finally, through various experiments, we show that the proposed method effectively and efficiently works in a large amount of multi-dimensional data.
Search
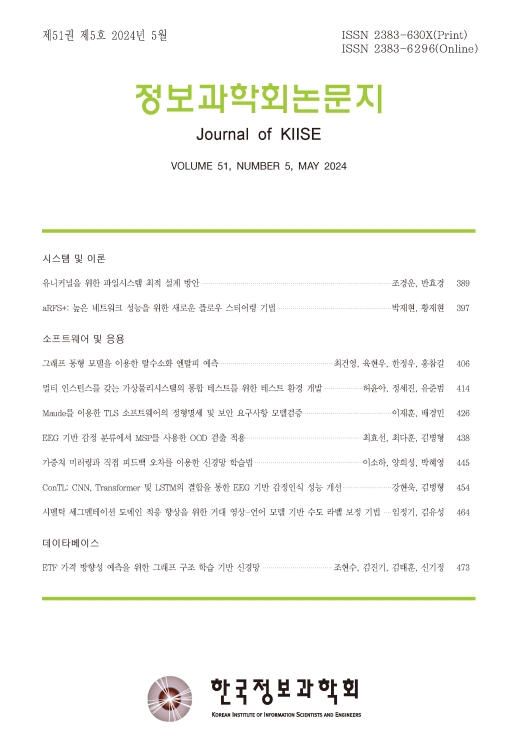
Journal of KIISE
- ISSN : 2383-630X(Print)
- ISSN : 2383-6296(Electronic)
- KCI Accredited Journal
Editorial Office
- Tel. +82-2-588-9240
- Fax. +82-2-521-1352
- E-mail. chwoo@kiise.or.kr