Digital Library[ Search Result ]
Enhanced Image Harmonization Scheme Using LAB Color Space-based Loss Function and Data Preprocessing
Doyeon Kim, Eunbeen Kim, Hyeonwoo Kim, Eenjun Hwang
http://doi.org/10.5626/JOK.2024.51.8.729
Image composition, which involves combining the background and foreground from different images to create a new image, is a useful technique in image editing. However, it often results in awkward images due to differences in brightness and color tones between the background and foreground. Image harmonization techniques aim to reduce this incongruity and have gained significant attention in the field of image editing. These techniques allow for realistic matching of color tones between the foreground and background. Existing deep learning models for image harmonization have shown promise in achieving harmonization performance through the use of large-scale training datasets. However, these models tend to exhibit poor generalization performance when the loss function does not effectively consider brightness or when the dataset has a biased brightness distribution. To address these issues, we propose an image harmonization scheme that is robust to variations in brightness. This scheme incorporates an LAB color space-based loss function, which explicitly calculates the brightness of a given image, and an LAB color space-based preprocessing scheme to create a dataset with a balanced brightness distribution. Experimental results on public image datasets demonstrate that the proposed scheme exhibits robust harmonization performance under various brightness conditions.
An Image Harmonization Method with Improved Visual Uniformity of Composite Images in Various Lighting Colors
Doyeon Kim, Jonghwa Shim, Hyeonwoo Kim, Changsu Kim, Eenjun Hwang
http://doi.org/10.5626/JOK.2024.51.4.345
Image composition is a technique that creates a composite image by arranging foreground objects extracted from other images onto a background image. To improve the visual uniformity of the composite images, deep learning-based image harmonization techniques that adjust the lighting and color of foreground objects to match the background image have been actively proposed recently. However, existing techniques have limited performance in visual uniformity because they adjust colors only for the lighting color distribution of the dataset used for training. To address this problem, we propose a novel image harmonization scheme that has robust performance for various lighting colors. First, iHColor, a new dataset composed of various lighting color distributions, is built through data preprocessing. Then, a pre-trained GAN-based Harmonization model is fine-tuned using the iHColor dataset. Through experiments, we demonstrate that the proposed scheme can generate harmonized images with better visual uniformity than existing models for various lighting colors.
A Twitter News-Classification Scheme Using Semantic Enrichment of Word Features
Seonmi Ji, Jihoon Moon, Hyeonwoo Kim, Eenjun Hwang
http://doi.org/10.5626/JOK.2018.45.10.1045
Recently, with the popularity of Twitter as a news platform, many news articles are generated, and various kinds of information and opinions about them spread out very fast. But since an enormous amount of Twitter news is posted simultaneously, users have difficulty in selectively browsing for news related to their interests. So far, many works have been conducted on how to classify Twitter news using machine learning and deep learning. In general, conventional machine learning schemes show data sparsity and semantic gap problems, and deep learning schemes require a large amount of data. To solve these problems, in this paper, we propose a Twitter news-classification scheme using semantic enrichment of word features. Specifically, we first extract the features of Twitter news data using the Vector Space Model. Second, we enhance those features using DBpedia Spotlight. Finally, we construct a topic-classification model based on various machine learning techniques and demonstrate by experiments that our proposed model is more effective than other traditional methods.
Search
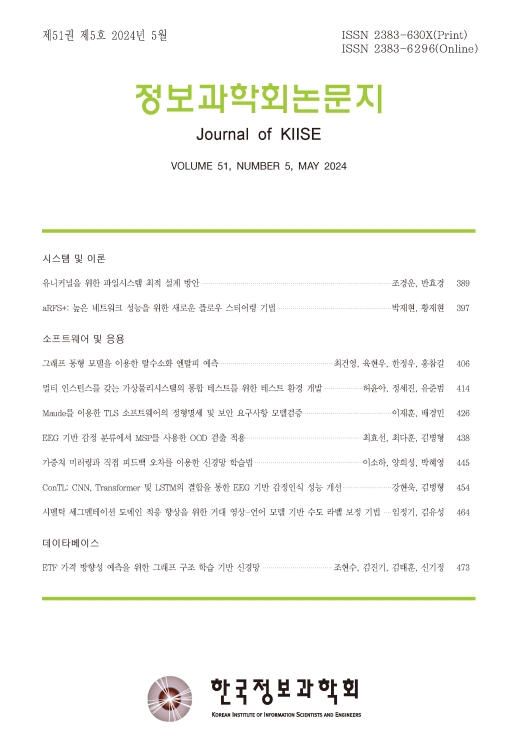
Journal of KIISE
- ISSN : 2383-630X(Print)
- ISSN : 2383-6296(Electronic)
- KCI Accredited Journal
Editorial Office
- Tel. +82-2-588-9240
- Fax. +82-2-521-1352
- E-mail. chwoo@kiise.or.kr