Digital Library[ Search Result ]
Graph Structure Learning: Reflecting Types of Relationships between Sensors in Multivariate Time Series Anomaly Detection
http://doi.org/10.5626/JOK.2024.51.3.236
Sensors are used to monitor systems in various fields, such as water treatment systems and smart factories. Anomalies in the system can be detected by analyzing multivariate time series consisting of sensor data. To efficiently detect anomalies, information about the relationships between sensors is required, but this information is generally difficult to obtain. To solve this problem, the previous work used sensor data to identify relationships between sensors, which were then represented using a graph structure. However, in this process, the graph structure only reflects the presence of relationships between sensors, not the types of relationships between sensors. In this pap er, we considered the types of relationships between sensors in graph structure learning and analyzed multivariate time series to detect anomalies in the system. Experiments show that improving detection accuracy in graph structure learning for multivariate time series anomaly detection involves taking into account the different kinds of relationships among sensors.
Knowledge Graph Embedding for Link Prediction using Node-Link Interaction-based Graph Attention Networks
http://doi.org/10.5626/JOK.2022.49.7.555
Knowledge graphs are structures that express knowledge in the real world in the form of nodes and links-based triple form. These knowledge graphs are incomplete and many embedding techniques have been studied to effectively represent nodes and links in low-dimensional vector spaces to find other missing relationships. Recently, many neural network-based knowledge graph link prediction methods have been studied. However existing models consider nodes and links independently when determining the importance of a triple to a node which makes it difficult to reflect the interaction between nodes and links. In this paper, we propose an embedding method that will be used to analyze the importance of triple units by simultaneously considering nodes and links using composition operators, and at the same time prove that the model outperforms other methods in knowledge graph link prediction.
Search
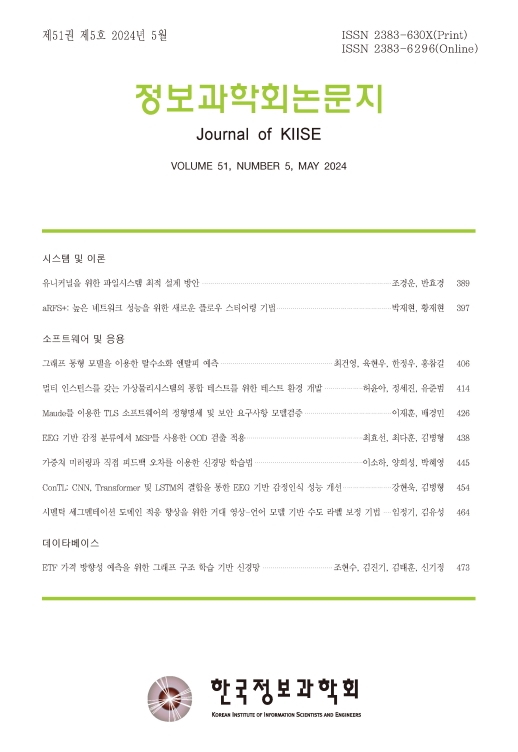
Journal of KIISE
- ISSN : 2383-630X(Print)
- ISSN : 2383-6296(Electronic)
- KCI Accredited Journal
Editorial Office
- Tel. +82-2-588-9240
- Fax. +82-2-521-1352
- E-mail. chwoo@kiise.or.kr