Digital Library[ Search Result ]
Deep Learning-based Models for Disaster Situation Awareness and Response Support
Eunjung Kwon, Minjung Lee, Hyuinho Park, Kyu-Chul Lee
http://doi.org/10.5626/JOK.2023.50.8.712
This paper is a study on decision support models for recognizing and responding to disaster situations by the control room receptionist who performs the 119-report reception work, which is directly related to the lives and properties of the people. To provide prompt, accurate, and effective first responders to emergency reports, it is essential to systematically respond according to the received situation from the beginning of the report. However, there are limitations in making decisions based on the individual capabilities of the 119 dispatcher in the face of various reports and frequently changing field conditions. Therefore, this paper proposes a deep learning-based disaster situation awareness model and a response support model that apply to the report reception work based on the 119 situation management standard manual. Lastly, we confirm the validity of the proposed method through experiments.
Improvement of the Classification Model Performance in 119-Emergency Report Data
Eunjung Kwon, Hyuinho Park, Sungwon Byon, Kyuchul Lee
http://doi.org/10.5626/JOK.2022.49.1.89
This paper presents a study of the text classification model to provide optimal response information for each disaster situation with respect to the report content recorded by the receiver in the process of receiving the 119 emergency report. A text classification model that receives a sentence and classifies it into a category is a widely used technique in the field of natural language processing. This study defined the rules for using augmented learning data to improve the performance of the text classification model through supervised learning, and confirmed the performance of the classification model using the augmented learning data through experiments. Through this study, the possibility of extension for improving the performance of the text classification model that is input as the report contents for each emergency situation, such as disease, traffic accident, and injury, was suggested.
Search
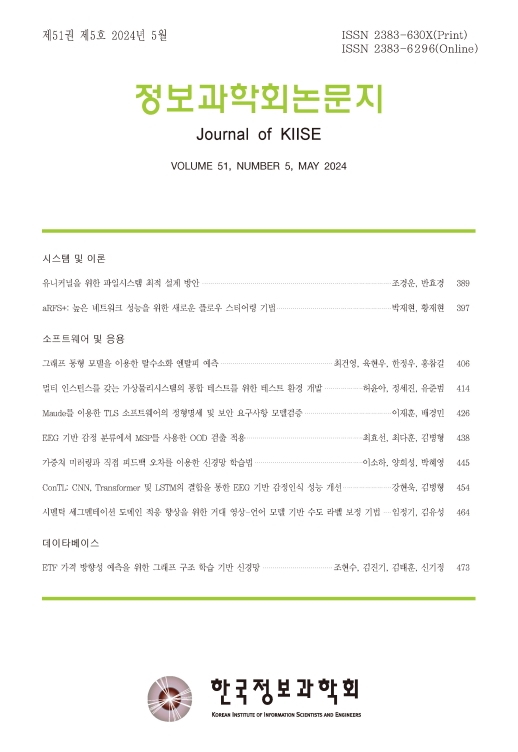
Journal of KIISE
- ISSN : 2383-630X(Print)
- ISSN : 2383-6296(Electronic)
- KCI Accredited Journal
Editorial Office
- Tel. +82-2-588-9240
- Fax. +82-2-521-1352
- E-mail. chwoo@kiise.or.kr