Digital Library[ Search Result ]
FedGC: Global Consistency Regularization for Federated Semi-supervised Learning
http://doi.org/10.5626/JOK.2022.49.12.1108
Recently, in the field of artificial intelligence, methods of learning neural network models in distributed environments that use sufficient data and hardware have been actively studied. Among them, federated learning, which guarantees privacy preservation without sharing data, has been a dominant scheme. However, existing federated learning methods assume supervised learning using only labeled data. Since labeling costs are incurred for supervised learning, the assumption that only label data exists in the clients is unrealistic. Therefore, this study proposes a federated semi-supervised learning method using both labeled data and unlabeled data, considering a more realistic situation where only labeled data exists on the server and unlabeled data on the client. We designed a loss function considering consistency regularization between the output distributions of the server and client models and analyzed how to adjust the influence of consistency regularization. The proposed method improved the performance of existing semi-supervised learning methods in federated learning settings, and through additional experiments, we analyzed the influence of the loss term and verified the validity of the proposed method.
Search
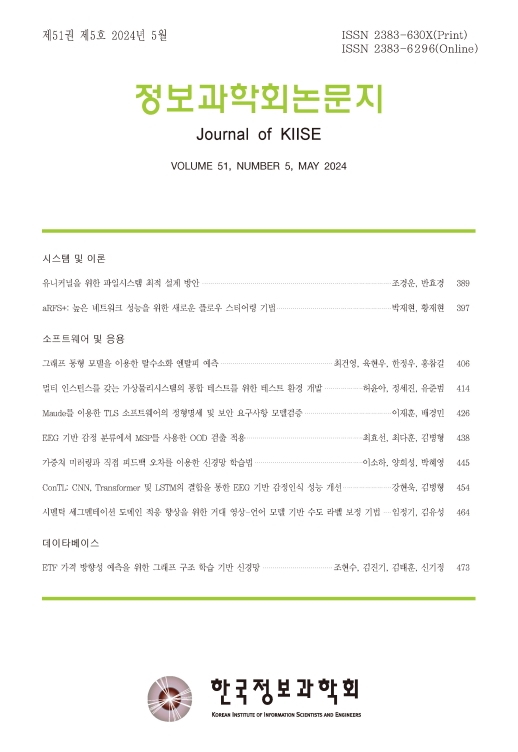
Journal of KIISE
- ISSN : 2383-630X(Print)
- ISSN : 2383-6296(Electronic)
- KCI Accredited Journal
Editorial Office
- Tel. +82-2-588-9240
- Fax. +82-2-521-1352
- E-mail. chwoo@kiise.or.kr