Digital Library[ Search Result ]
SASRec vs. BERT4Rec: Performance Analysis of Transformer-based Sequential Recommendation Models
Hye-young Kim, Mincheol Yoon, Jongwuk Lee
http://doi.org/10.5626/JOK.2024.51.4.352
Sequential recommender systems extract interests from user logs and use them to recommend items the user might like next. SASRec and BERT4Rec are widely used as representative sequential recommendation models. Existing studies have utilized these two models as baselines in various studies, but their performance is not consistent due to differences in experimental environments. This research compares and analyzes the performance of SASRec and BERT4Rec on six representative sequential recommendation datasets. The experimental result shows that the number of user-item interactions has the largest impact on BERT4Rec training, which in turn leads to the performance difference between the two models. Furthermore, this research finds that the two learning methods, which are widely utilized in sequential recommendation settings, can also have different effects depending on the popularity bias and sequence length. This shows that considering dataset characteristics is essential for improving recommendation performance.
Search
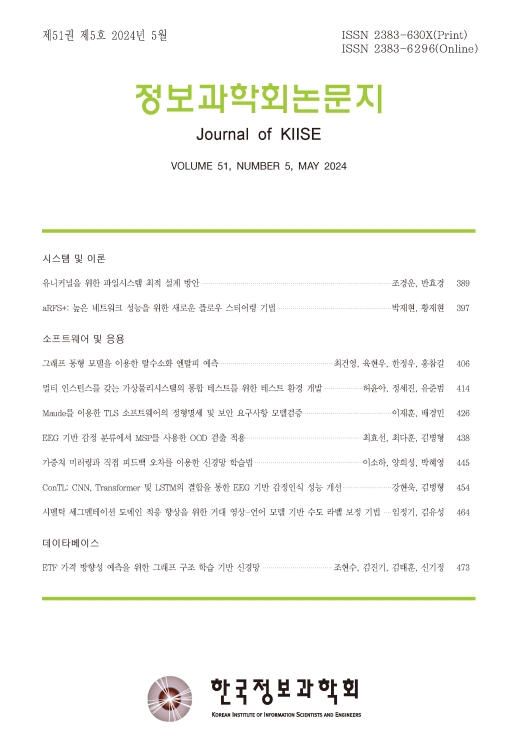
Journal of KIISE
- ISSN : 2383-630X(Print)
- ISSN : 2383-6296(Electronic)
- KCI Accredited Journal
Editorial Office
- Tel. +82-2-588-9240
- Fax. +82-2-521-1352
- E-mail. chwoo@kiise.or.kr