Digital Library[ Search Result ]
Improving the Quality of Generating Imbalance Data in GANs through an Exhaustive Contrastive Learning Method
Hyeonjun Shin, Sangbaek Lee, Kyuchul Lee
http://doi.org/10.5626/JOK.2023.50.4.295
As the performance of deep learning algorithms has improved, they are being used as a way to solve various problems in the real world. In the case of data that reflect the real world, imbalance data may occur depending on the frequency of occurrence of events or the difficulty of collection. Data with an inconsistent number of classes that make up the data are called imbalance data, and in particular, it is difficult to learn the minority classes with relatively little data through Deep Learning algorithms. Recently, Generative Adversarial Nets (GANs) have been applied as a method for data augmentation, and self-supervised learning-based pre-learning has been proposed for minority class learning. However, because class information of imbalance data is utilized in the process of learning the Generative Model, the quality of generated data is poor due to poor learning of minority classes. To solve this problem, this paper proposes a similarity-based exhaustive contrast learning method. The proposed method is quantitatively evaluated through the Frechet Inception Distance (FID) and Inception Score (IS). The method proposed in this paper confirmed the performance improvement of the Frechet Inception Distance of 16.32 and the Inception Score of 0.38, as compared to the existing method.
Improvement of the Classification Model Performance in 119-Emergency Report Data
Eunjung Kwon, Hyuinho Park, Sungwon Byon, Kyuchul Lee
http://doi.org/10.5626/JOK.2022.49.1.89
This paper presents a study of the text classification model to provide optimal response information for each disaster situation with respect to the report content recorded by the receiver in the process of receiving the 119 emergency report. A text classification model that receives a sentence and classifies it into a category is a widely used technique in the field of natural language processing. This study defined the rules for using augmented learning data to improve the performance of the text classification model through supervised learning, and confirmed the performance of the classification model using the augmented learning data through experiments. Through this study, the possibility of extension for improving the performance of the text classification model that is input as the report contents for each emergency situation, such as disease, traffic accident, and injury, was suggested.
Ontology Construction for Interoperability and Scalability of Human-Object Interaction Datasets
Aryoung Kim, Sangbaek Lee, Kyuchul Lee
http://doi.org/10.5626/JOK.2021.48.8.947
Human behavior can be expressed through actions and objects. In order to understand the human behavior, the interaction with objects must be considered, as well as human behavior. The Human-Object Interaction (HOI) can be expressed in rich and varied ways. As detailed representations were required, large-scale datasets were generated. However, as they were written in different file formats and expression methods, decreasing interoperability between each other made it difficult to add, modify, or delete new objects or relationships. In this paper, we constructed a HOI ontology which can be utilized in the field of HOI research. We designed ontology to express the relationship between humans and objects. We then developed a method of instance generation and extension for interoperability support between HOI datasets. The design of the ontology allowed clear identification and expression of extracted information. Moreover, the extension method provided semantic interoperability so that new objects or relationships could be continuously extended to the HOI ontology.
Search
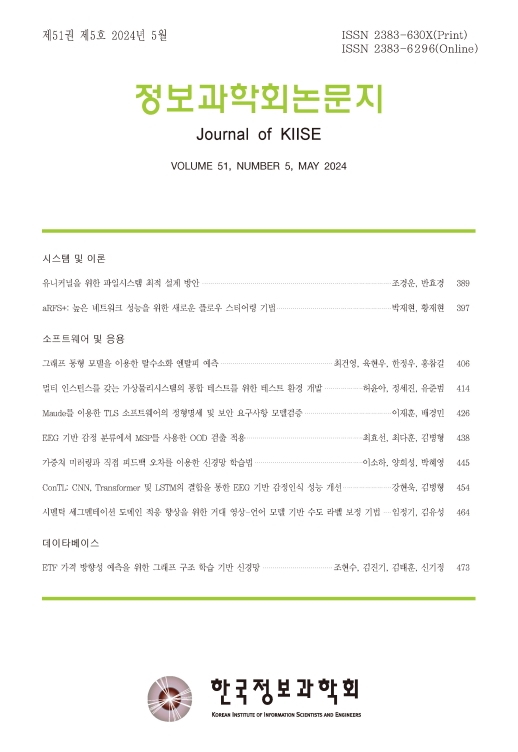
Journal of KIISE
- ISSN : 2383-630X(Print)
- ISSN : 2383-6296(Electronic)
- KCI Accredited Journal
Editorial Office
- Tel. +82-2-588-9240
- Fax. +82-2-521-1352
- E-mail. chwoo@kiise.or.kr