Digital Library[ Search Result ]
Improvement of Prostate Cancer Aggressiveness Prediction Based on the Deep Learning Model Using Size Normalization and Multiple Loss Functions on Multi-parametric MR Images
Yoon Jo Kim, Julip Jung, Sung Il Hwang, Helen Hong
http://doi.org/10.5626/JOK.2023.50.10.866
Prostate cancer is the second most common cancer in men worldwide, and it is essential to predict the aggressiveness of prostate cancer because the recurrence rate and the effectiveness of treatment vary depending on the aggressiveness. This study enhances the information on small tumors by applying size normalization to predict the aggressiveness of prostate cancer in multi-parametric MR imaging. Additionally, we propose the use of multiple loss functions to distinguish tumors with different aggressiveness while having a similar visual appearance. Experimental results show that the proposed model trained with size-normalized ADC maps achieves an accuracy of 76.28%, sensitivity of 76.81%, specificity of 75.86%, and an AUC of 0.77. Moreover, compared to the tumor-centered ADC maps, size-normalized ADC maps demonstrate improved performance in tumors smaller than 1.5 cm, with an accuracy of 76.47%, sensitivity of 90.91%, and specificity of 69.57%, corresponding to a significant improvement of 17.65%, 27.27%, and 13.05% respectively.
Automatic Classification of Pneumonia Based on Ensemble Deep Learning Model Using Intensity Normalization and Multiscale Lung-Focused Patches on Chest X-Ray Images
Yoon Jo Kim, Jinseo An, Helen Hong
http://doi.org/10.5626/JOK.2022.49.9.677
It is difficult to classify normal and pneumonia in pediatric chest X-ray (CXR) images due to irregular intensity values. In addition, deep learning model has a limitation in that it can misclassify CXR by incorrectly focusing on the outer part of the lung. This study proposed an automatic classification of pneumonia based on ensemble deep learning model using three intensity normalizations and multiscale lung-focused patches on CXR images. First, to correct for irregular intensity values in internal lungs, three intensity normalization methods were performed respectively. Second, to focus on internal lungs, regions of interest were extracted by segmenting lung regions. Third, multiscale lung-focused patches were extracted to train the characterization of pneumonia. Finally, ensemble modeling with attention module was performed to improve the classification performance. In the experiment, the method using large patches of CLAHE images showed an accuracy of 92%, which was 5% higher than that of original images. Furthermore, the proposed method using an ensemble of large and middle patches showed the best performance with an accuracy of 93%.
Search
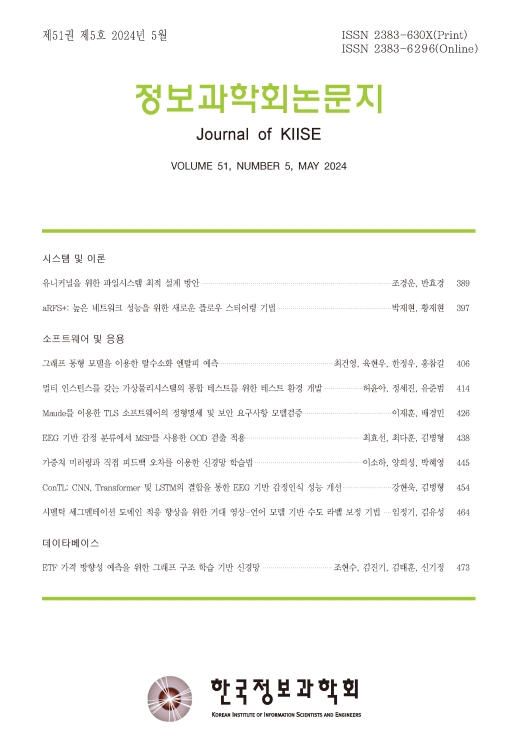
Journal of KIISE
- ISSN : 2383-630X(Print)
- ISSN : 2383-6296(Electronic)
- KCI Accredited Journal
Editorial Office
- Tel. +82-2-588-9240
- Fax. +82-2-521-1352
- E-mail. chwoo@kiise.or.kr