Digital Library[ Search Result ]
Activity Prediction from Sensor Data using Convolutional Neural Networks and an Efficient Compression Method
Woojeong Jin, Dongjin Choi, Youngjin Kim, U Kang
http://doi.org/10.5626/JOK.2018.45.6.564
The identification of the number of occupants and their activities using the IoT system in a building is an important task to improve the power efficiency and reduce the cost of using smart cooling/heating systems. In the actual building management system, it is possible to use equipment such as a camera to understand the current situation in the room, and to directly determine the number of occupants and their types of behavior. However, identifying the number of people and behavior types in this way is inefficient and requires a large amount of storage space for data. In this study, indoor sensor data were collected using an infrared Grid-Eye sensor and noise sensor. Based on this data, we also propose a deep learning model that captures the number of participants and behavior patterns and a deep learning model that considers the temporal characteristics of data. The proposed model identifies the number of people with an accuracy of about 95.3% and human activities with an accuracy of 90.9%. We also propose a method to reduce the storage space while minimizing the loss of accuracy using truncated SVD.
Event Cognition-based Daily Activity Prediction Using Wearable Sensors
Chung-Yeon Lee, Dong Hyun Kwak, Beom-Jin Lee, Byoung-Tak Zhang
Learning from human behaviors in the real world is essential for human-aware intelligent systems such as smart assistants and autonomous robots. Most of research focuses on correlations between sensory patterns and a label for each activity. However, human activity is a combination of several event contexts and is a narrative story in and of itself. We propose a novel approach of human activity prediction based on event cognition. Egocentric multi-sensor data are collected from an individual’s daily life by using a wearable device and smartphone. Event contexts about location, scene and activities are then recognized, and finally the users’’ daily activities are predicted from a decision rule based on the event contexts. The proposed method has been evaluated on a wearable sensor data collected from the real world over 2 weeks by 2 people. Experimental results showed improved recognition accuracies when using the proposed method comparing to results directly using sensory features.
Search
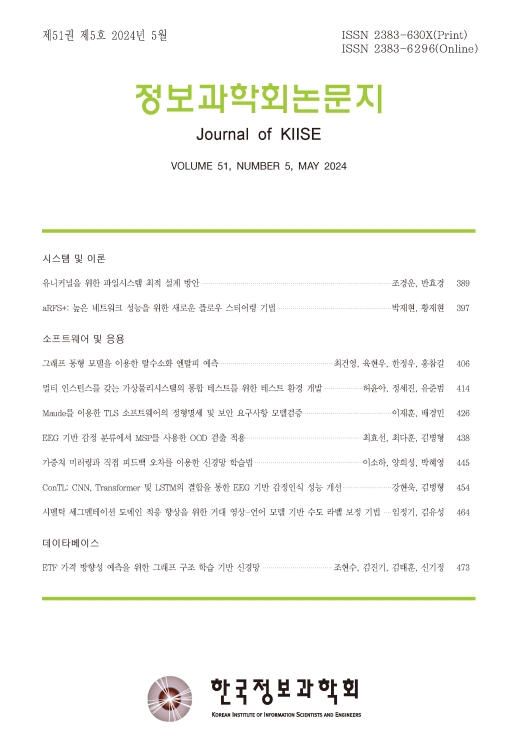
Journal of KIISE
- ISSN : 2383-630X(Print)
- ISSN : 2383-6296(Electronic)
- KCI Accredited Journal
Editorial Office
- Tel. +82-2-588-9240
- Fax. +82-2-521-1352
- E-mail. chwoo@kiise.or.kr