Study on the Evaluation of Embedding Models in the Natural Language Processing
Vol. 52, No. 2, pp. 141-151, Feb. 2025

-
Retrieval-Augmented Generation embedding models Natural Language Processing generative language model
Abstract
Statistics
Cumulative Counts from November, 2022
Multiple requests among the same browser session are counted as one view. If you mouse over a chart, the values of data points will be shown.
Multiple requests among the same browser session are counted as one view. If you mouse over a chart, the values of data points will be shown.
|
Cite this article
[IEEE Style]
H. Kang, "Study on the Evaluation of Embedding Models in the Natural Language Processing," Journal of KIISE, JOK, vol. 52, no. 2, pp. 141-151, 2025. DOI: 10.5626/JOK.2025.52.2.141.
[ACM Style]
Hanhoon Kang. 2025. Study on the Evaluation of Embedding Models in the Natural Language Processing. Journal of KIISE, JOK, 52, 2, (2025), 141-151. DOI: 10.5626/JOK.2025.52.2.141.
[KCI Style]
강한훈, "자연어처리 분야에서의 임베딩 모델 평가 연구," 한국정보과학회 논문지, 제52권, 제2호, 141~151쪽, 2025. DOI: 10.5626/JOK.2025.52.2.141.
[Endnote/Zotero/Mendeley (RIS)] Download
[BibTeX] Download
Search
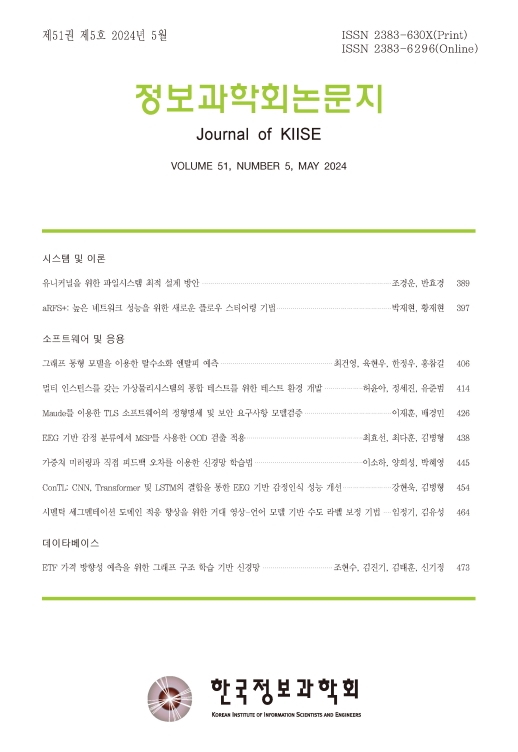
Journal of KIISE
- ISSN : 2383-630X(Print)
- ISSN : 2383-6296(Electronic)
- KCI Accredited Journal
Editorial Office
- Tel. +82-2-588-9240
- Fax. +82-2-521-1352
- E-mail. chwoo@kiise.or.kr