Digital Library[ Search Result ]
Ontology and CNN-based Inference of the Threat Relationship Between UAVs and Surrounding Objects
MyungJoong Jeon, MinHo Lee, HyunKyu Park, YoungTack Park, Hyung-Sik Yoon, Yun-Geun Kim
http://doi.org/10.5626/JOK.2020.47.4.404
The technology that identifies the relationship between surrounding objects and recognizes the situation is considered as an important and necessary technology in various areas. Numerous methodologies are being studied for this purpose. Most of the studies have solved the problem by building the domain knowledge into ontology for reasoning of situation awareness. However, based on the existing approach; it is difficult to deal with new situations in the absence of domain experts due to the dependency of experts on relevant domain knowledge. In addition, it is difficult to build the knowledge to infer situations that experts have not considered. Therefore, this study proposes a model for using ontology and CNN for reasoning of the relationships between UAVs and surrounding objects to solve the existing problems. Based on the assumption that the accuracy of ontology reasoning is insufficient, first, the reasoning was performed using the information from the detected surrounding objects. Later, the results of ontology reasoning are revised by CNN inference. Due to the limitations of actual data acquisition, data generator was built to generate data similar to real data. For evaluation of this study, two models of relationships between two objects were built and evaluated; both the models showed over 90% accuracy.
An Approach for Recognition of Elderly Living Patterns Based on Event Calculus Using Percept Sequence
Hyun-Kyu Park, Young-Tack Park
http://doi.org/10.5626/JOK.2019.46.11.1149
This paper proposes a method for recognizing the intentions of human activity based on percept sequences that represent the activities of daily living (ADL) in a residential space. Based on the activity intention ontology, which represents actions and poses related to human activity intentions, the proposed method identifies the intention of a human activity by using event calculus when a percept sequence is entered. Based on the action intent identified, frequency and pattern analysis of the action intention is used to characterize the lifestyle patterns of the elderly. The intentions of everyday behavior occurring in an elderly living space are complex, and it is difficult to recognize the pattern of life through these intentions, which makes it difficult to recognize the intention of a complex occurrence. To solve these problems, this paper constructs an ontology of percept sequences expressed as daily behavioral information, and makes inferences to help recognize activity intent based on event calculus. When evaluating the techniques proposed in this paper, the results of the act intention cognition experiment based on the perceptual information recorded showed 84% precision and 85% recall.
An Autonomous Threat Situation Awareness System for UAV based on Ontology
MyungJoong Jeon, HyunKyu Park, YoungTack Park, Hyung-Sik Yoon, Yun-Geun Kim
http://doi.org/10.5626/JOK.2019.46.10.1044
An autonomous threat situational awareness system is necessary for Unmanned Aerial Vehicles(UAVs) in a variety of fields. Although various of approaches to autonomous threat situational awareness have been proposed, most of them involved reasoning of the semantic information of the object. Therefore, in this paper, based on the existing semantic information of an object, we propose a method to achieve threat situational awareness for a UAV based on reasoning of the relationship between the objects. In this paper, there are three main ways that are used to recognize a threat to a UAV: First, information on the recognized objects is expressed using an LOD(Level of Detail)-based grid map. Second, the concepts of objects around the UAV are defined as ontology while the relationships and situations between objects are defined as SWRL(Semantic Web Rule Language). Third, through the ontology reasoning, the simulator visualizes the recognition of the relationships of objects and threat situations for the UAV.
A UAV Situational Awareness Method through the Threat-Related Relation Reasoning between UAV and Surrounding Objects
Seok-Hyun Bae, Myung-Joong Jeon, Hyun-Kyu Park, Young-Tack Park, Hyung-Sik Yoon, Yun-Geun Kim
http://doi.org/10.5626/JOK.2019.46.2.141
As the technological capabilities of UAV(Unmanned Aerial Vehicles) improves, studies are being carried out to intelligently analyze and understand the situation of UAV in order to gain access to the target area while recognizing and avoiding various risks. To achieve the mission of UAV, it is necessary to judge the situation accurately and quickly. To do this, this paper proposes ways to infer the threat-related relationship between an UAV and perceived surrounding objects through a 3 step approach and provide abstract information about the situation of UAV. The first step is to instantiate the object data recognized by UAV to be utilized for ontology and rule-based reasoning. The second step is to define the priority of instantiated object data and to infer the threat-related relationship between them. Finally, recognizing the situation through the relationship inference that takes into account the association between current and past inferred relationships. To evaluation the performance of the proposed method, a virtual UAV environment simulator was built and tested the data 1,000 times that were randomly generated through five sequential UAV moving point paths. Eight kinds of objects could be recognized in UAV path and ten kinds of relationships can be inferred. Overall performance of situation Awareness was an average of 91 percent.
Incorrect Triple Detection Using Knowledge Base Embedding and Relation Model
Ji-Hun Hong, Hyun-Young Choi, Wan-Gon Lee, Young-Tack Park
http://doi.org/10.5626/JOK.2019.46.2.131
Recently, with the increase of the amount of information due to the development of the Internet, there has been an increased interest in research using a large-capacity knowledge base. Additionally, studies are being conducted to complete the knowledge base as it uses become widely used in various studies. However, there has been lack of research to detect error triples in the knowledge base. This paper, we proposes the embedding of an algorithm to detect the error triple in the knowledge base, the utilization of the clustered embedding model and the four relational models, which are typical algorithms of triple classification. Additionally, a relation ensemble model was generated using the results of the single embedding models and the embedding ensemble model similarly generated using the results of the single embedding models. The error triple detection results were then compared and measured through the model verification indexes.
Partial Embedding Approach for Knowledge Completion
Wan-Gon Lee, Batselem Jagvaral, Ji-Hun Hong, Hyun-Young Choi, Young-Tack Park
http://doi.org/10.5626/JOK.2018.45.11.1168
Knowledge graphs are large networks that describe real world entities and their relationships with triples. Most of the knowledge graphs are far from being complete, and many previous studies have addressed this problem using low dimensional graph embeddings. Such methods assume that knowledge graphs are fixed and do not change. However, real-world knowledge graphs evolve at a rapid pace with the addition of new triples.Repeated retraining of embedding models for the entire graph is computationally expensive and impractical. In this paper, we propose a partial embedding method for partial completion of evolving knowledge graphs. Our method employs ontological axioms and contextual information to extract relations of interest and builds entity and relation embedding models based on instances of such relations. Our experiments demonstrated that the proposed partial embedding method can produce comparable results on knowledge graph completion with state-of-the-art methods while significantly reducing the computation time of entity and relation embeddings by 49%–90% for the Freebase and WiseKB datasets.
Integrated Explanation System for a Scalable Data based on SPARQL Results
MyungJoong Jeon, HyunKyu Park, YoungTack Park
http://doi.org/10.5626/JOK.2018.45.10.1004
Recently, there has been an increasing demand for an explanation of query results in a variety of QA systems and expert systems. However, the systems being studied today only focus on the scalable query processing. Therefore, this paper proposes an integrated system that explains the causal relationship to the query results based on large volumes of retrievable data. The system uses a distributed rule-based SWRL engine for reasoning about large amounts of knowledge. And in this case uses evidence of reasoning as input for a distributed ATMS to express the structure of the causal relationship. Finally, after obtaining the answers using SPARQLGX, and a scalable SPARQL query processor, this system explains the evidence of answers using a reference to the previously established dependency structure. The evaluation of the proposed explanation system used the benchmark data(Lehigh University Benchmark) and used 14 test queries provided by the LUBM for evaluating the response time and explanation time in this case.
Knowledge Completion Modeling using Knowledge Base Embedding
Hyun-Young Choi, Ji-Hun Hong, Wan-Gon Lee, Batselem Jagvaral, Myung-Joong Jeon, Hyun-Kyu Park, Young-Tack Park
http://doi.org/10.5626/JOK.2018.45.9.895
In recent years, a number of studies have been conducted for the purpose of automatically building a knowledge base that is based on web data. However, due to the incomplete nature of web data, there can be missing data or a lack of connections among the data entities that are present. In order to solve this problem, recent studies have proposed methods that train a model to predict this missing data through an artificial neural network based on natural language embedding, but there is a drawback to embedding entities. In practice, natural language corpus is not present in many knowledge bases. Therefore, in this paper, we propose a knowledge completion method that converts the knowledge base of RDF data into an RDF-sentence and uses embedding to create word vectors. We conducted a triple classification experiment in order to measure the performance of the proposed method. The proposed method was then compared with existing NTN models, and on average, 15% accuracy was obtained. In addition, we obtained 88%accuracy by applying the proposed method to the Korean knowledge base known as WiseKB.
An Approach to a Learning Prediction Model for Recognition of Daily Life Pattern based on Event Calculus
Seok-Hyun Bae, Sung-hyuk Bang, Hyun-Kyu Park, Myung-Joong Jeon, Je-Min Kim, Young-Tack Park
http://doi.org/10.5626/JOK.2018.45.5.466
Several studies have been conducted on data analysis and predicting results with the advance of machine learning algorithms. Still, there are many problems of cleaning the noise of the real-life dataset, which is disturbing a clear recognition on complex patterns of human intention. To overcome this limitation, this paper proposes an event calculus methodology with 3 additional steps for the recognition of human intention: intention reasoning, conflict resolution, and noise reduction. Intention reasoning identifies the intention of the living pattern time-series data. In conflict resolution, existing ongoing intentions and inferred intention are checked by a conflict graph, so that the intentions that can occur in parallel are inferred. Finally, for noise reduction, the inferred intention from the noise of living pattern data is filtered by the history of fluent. For the evaluation of the event calculus module, this paper also proposes data generation methodology based on a gaussian mixture model and heuristic rules. The performance estimation was conducted with 300 sequential instances with 5 intentions that were observed for 13 hours. An accuracy of 89.3% was achieved between the probabilistic model and event calculus module.
Approach for Learning Intention Prediction Model based on Recurrent Neural Network
Sung-hyuk Bang, Seok-Hyun Bae, Hyun-Kyu Park, Myung-Joong Jeon, Je-Min Kim, Young-Tack Park
http://doi.org/10.5626/JOK.2018.45.4.360
Several studies have been conducted on human intention prediction with the help of machine learning models. However, these studies have indicated a fundamental shortcoming of machine learning models since they are unable to reflect a long span of past information. To overcome this limitation, this paper proposes a human intention prediction model based on a recurrent neural network(RNN). For performing predictions, the RNN model classifies the patterns of time-series data by reflecting previous sequence patterns of the time-series data. For performing intention prediction using the proposed model, an RNN model was trained to classify predefined intentions by using attributes such as time, location, activity and detected objects in a house. Each RNN node is composed of a long short-term memory cell to solve the long term dependency problem. To evaluate the proposed intention prediction model, a data generator based on the weighted-graph structure has been developed for generating data on a daily basis. By incorporating 23,000 data instances for training and testing the proposed intention prediction model, a prediction accuracy value of 90.52% was achieved.
Search
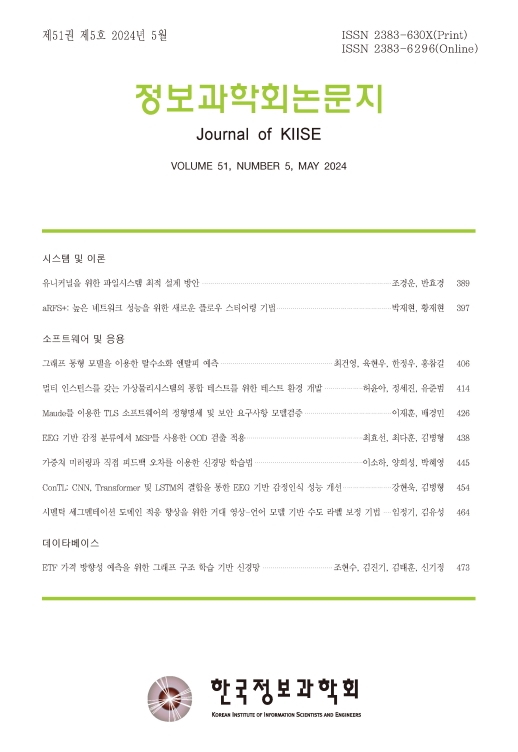
Journal of KIISE
- ISSN : 2383-630X(Print)
- ISSN : 2383-6296(Electronic)
- KCI Accredited Journal
Editorial Office
- Tel. +82-2-588-9240
- Fax. +82-2-521-1352
- E-mail. chwoo@kiise.or.kr