Digital Library[ Search Result ]
Ontology and CNN-based Inference of the Threat Relationship Between UAVs and Surrounding Objects
MyungJoong Jeon, MinHo Lee, HyunKyu Park, YoungTack Park, Hyung-Sik Yoon, Yun-Geun Kim
http://doi.org/10.5626/JOK.2020.47.4.404
The technology that identifies the relationship between surrounding objects and recognizes the situation is considered as an important and necessary technology in various areas. Numerous methodologies are being studied for this purpose. Most of the studies have solved the problem by building the domain knowledge into ontology for reasoning of situation awareness. However, based on the existing approach; it is difficult to deal with new situations in the absence of domain experts due to the dependency of experts on relevant domain knowledge. In addition, it is difficult to build the knowledge to infer situations that experts have not considered. Therefore, this study proposes a model for using ontology and CNN for reasoning of the relationships between UAVs and surrounding objects to solve the existing problems. Based on the assumption that the accuracy of ontology reasoning is insufficient, first, the reasoning was performed using the information from the detected surrounding objects. Later, the results of ontology reasoning are revised by CNN inference. Due to the limitations of actual data acquisition, data generator was built to generate data similar to real data. For evaluation of this study, two models of relationships between two objects were built and evaluated; both the models showed over 90% accuracy.
An Autonomous Threat Situation Awareness System for UAV based on Ontology
MyungJoong Jeon, HyunKyu Park, YoungTack Park, Hyung-Sik Yoon, Yun-Geun Kim
http://doi.org/10.5626/JOK.2019.46.10.1044
An autonomous threat situational awareness system is necessary for Unmanned Aerial Vehicles(UAVs) in a variety of fields. Although various of approaches to autonomous threat situational awareness have been proposed, most of them involved reasoning of the semantic information of the object. Therefore, in this paper, based on the existing semantic information of an object, we propose a method to achieve threat situational awareness for a UAV based on reasoning of the relationship between the objects. In this paper, there are three main ways that are used to recognize a threat to a UAV: First, information on the recognized objects is expressed using an LOD(Level of Detail)-based grid map. Second, the concepts of objects around the UAV are defined as ontology while the relationships and situations between objects are defined as SWRL(Semantic Web Rule Language). Third, through the ontology reasoning, the simulator visualizes the recognition of the relationships of objects and threat situations for the UAV.
A UAV Situational Awareness Method through the Threat-Related Relation Reasoning between UAV and Surrounding Objects
Seok-Hyun Bae, Myung-Joong Jeon, Hyun-Kyu Park, Young-Tack Park, Hyung-Sik Yoon, Yun-Geun Kim
http://doi.org/10.5626/JOK.2019.46.2.141
As the technological capabilities of UAV(Unmanned Aerial Vehicles) improves, studies are being carried out to intelligently analyze and understand the situation of UAV in order to gain access to the target area while recognizing and avoiding various risks. To achieve the mission of UAV, it is necessary to judge the situation accurately and quickly. To do this, this paper proposes ways to infer the threat-related relationship between an UAV and perceived surrounding objects through a 3 step approach and provide abstract information about the situation of UAV. The first step is to instantiate the object data recognized by UAV to be utilized for ontology and rule-based reasoning. The second step is to define the priority of instantiated object data and to infer the threat-related relationship between them. Finally, recognizing the situation through the relationship inference that takes into account the association between current and past inferred relationships. To evaluation the performance of the proposed method, a virtual UAV environment simulator was built and tested the data 1,000 times that were randomly generated through five sequential UAV moving point paths. Eight kinds of objects could be recognized in UAV path and ten kinds of relationships can be inferred. Overall performance of situation Awareness was an average of 91 percent.
Integrated Explanation System for a Scalable Data based on SPARQL Results
MyungJoong Jeon, HyunKyu Park, YoungTack Park
http://doi.org/10.5626/JOK.2018.45.10.1004
Recently, there has been an increasing demand for an explanation of query results in a variety of QA systems and expert systems. However, the systems being studied today only focus on the scalable query processing. Therefore, this paper proposes an integrated system that explains the causal relationship to the query results based on large volumes of retrievable data. The system uses a distributed rule-based SWRL engine for reasoning about large amounts of knowledge. And in this case uses evidence of reasoning as input for a distributed ATMS to express the structure of the causal relationship. Finally, after obtaining the answers using SPARQLGX, and a scalable SPARQL query processor, this system explains the evidence of answers using a reference to the previously established dependency structure. The evaluation of the proposed explanation system used the benchmark data(Lehigh University Benchmark) and used 14 test queries provided by the LUBM for evaluating the response time and explanation time in this case.
Knowledge Completion Modeling using Knowledge Base Embedding
Hyun-Young Choi, Ji-Hun Hong, Wan-Gon Lee, Batselem Jagvaral, Myung-Joong Jeon, Hyun-Kyu Park, Young-Tack Park
http://doi.org/10.5626/JOK.2018.45.9.895
In recent years, a number of studies have been conducted for the purpose of automatically building a knowledge base that is based on web data. However, due to the incomplete nature of web data, there can be missing data or a lack of connections among the data entities that are present. In order to solve this problem, recent studies have proposed methods that train a model to predict this missing data through an artificial neural network based on natural language embedding, but there is a drawback to embedding entities. In practice, natural language corpus is not present in many knowledge bases. Therefore, in this paper, we propose a knowledge completion method that converts the knowledge base of RDF data into an RDF-sentence and uses embedding to create word vectors. We conducted a triple classification experiment in order to measure the performance of the proposed method. The proposed method was then compared with existing NTN models, and on average, 15% accuracy was obtained. In addition, we obtained 88%accuracy by applying the proposed method to the Korean knowledge base known as WiseKB.
An Approach to a Learning Prediction Model for Recognition of Daily Life Pattern based on Event Calculus
Seok-Hyun Bae, Sung-hyuk Bang, Hyun-Kyu Park, Myung-Joong Jeon, Je-Min Kim, Young-Tack Park
http://doi.org/10.5626/JOK.2018.45.5.466
Several studies have been conducted on data analysis and predicting results with the advance of machine learning algorithms. Still, there are many problems of cleaning the noise of the real-life dataset, which is disturbing a clear recognition on complex patterns of human intention. To overcome this limitation, this paper proposes an event calculus methodology with 3 additional steps for the recognition of human intention: intention reasoning, conflict resolution, and noise reduction. Intention reasoning identifies the intention of the living pattern time-series data. In conflict resolution, existing ongoing intentions and inferred intention are checked by a conflict graph, so that the intentions that can occur in parallel are inferred. Finally, for noise reduction, the inferred intention from the noise of living pattern data is filtered by the history of fluent. For the evaluation of the event calculus module, this paper also proposes data generation methodology based on a gaussian mixture model and heuristic rules. The performance estimation was conducted with 300 sequential instances with 5 intentions that were observed for 13 hours. An accuracy of 89.3% was achieved between the probabilistic model and event calculus module.
Approach for Learning Intention Prediction Model based on Recurrent Neural Network
Sung-hyuk Bang, Seok-Hyun Bae, Hyun-Kyu Park, Myung-Joong Jeon, Je-Min Kim, Young-Tack Park
http://doi.org/10.5626/JOK.2018.45.4.360
Several studies have been conducted on human intention prediction with the help of machine learning models. However, these studies have indicated a fundamental shortcoming of machine learning models since they are unable to reflect a long span of past information. To overcome this limitation, this paper proposes a human intention prediction model based on a recurrent neural network(RNN). For performing predictions, the RNN model classifies the patterns of time-series data by reflecting previous sequence patterns of the time-series data. For performing intention prediction using the proposed model, an RNN model was trained to classify predefined intentions by using attributes such as time, location, activity and detected objects in a house. Each RNN node is composed of a long short-term memory cell to solve the long term dependency problem. To evaluate the proposed intention prediction model, a data generator based on the weighted-graph structure has been developed for generating data on a daily basis. By incorporating 23,000 data instances for training and testing the proposed intention prediction model, a prediction accuracy value of 90.52% was achieved.
SWAT: A Study on the Efficient Integration of SWRL and ATMS based on a Distributed In-Memory System
Myung-Joong Jeon, Wan-Gon Lee, Batselem Jagvaral, Hyun-Kyu Park, Young-Tack Park
http://doi.org/10.5626/JOK.2018.45.2.113
Recently, with the advent of the Big Data era, we have gained the capability of acquiring vast amounts of knowledge from various fields. The collected knowledge is expressed by well-formed formula and in particular, OWL, a standard language of ontology, is a typical form of well-formed formula. The symbolic reasoning is actively being studied using large amounts of ontology data for extracting intrinsic information. However, most studies of this reasoning support the restricted rule expression based on Description Logic and they have limited applicability to the real world. Moreover, knowledge management for inaccurate information is required, since knowledge inferred from the wrong information will also generate more incorrect information based on the dependencies between the inference rules. Therefore, this paper suggests that the SWAT, knowledge management system should be combined with the SWRL (Semantic Web Rule Language) reasoning based on ATMS (Assumption-based Truth Maintenance System). Moreover, this system was constructed by combining with SWRL reasoning and ATMS for managing large ontology data based on the distributed In-memory framework. Based on this, the ATMS monitoring system allows users to easily detect and correct wrong knowledge. We used the LUBM (Lehigh University Benchmark) dataset for evaluating the suggested method which is managing the knowledge through the retraction of the wrong SWRL inference data on large data.
Distributed In-Memory based Large Scale RDFS Reasoning and Query Processing Engine for the Population of Temporal/Spatial Information of Media Ontology
Wan-Gon Lee, Nam-Gee Lee, MyungJoong Jeon, Young-Tack Park
Providing a semantic knowledge system using media ontologies requires not only conventional axiom reasoning but also knowledge extension based on various types of reasoning. In particular, spatio-temporal information can be used in a variety of artificial intelligence applications and the importance of spatio-temporal reasoning and expression is continuously increasing. In this paper, we append the LOD data related to the public address system to large-scale media ontologies in order to utilize spatial inference in reasoning. We propose an RDFS/Spatial inference system by utilizing distributed memory-based framework for reasoning about large-scale ontologies annotated with spatial information. In addition, we describe a distributed spatio-temporal SPARQL parallel query processing method designed for large scale ontology data annotated with spatio-temporal information. In order to evaluate the performance of our system, we conducted experiments using LUBM and BSBM data sets for ontology reasoning and query processing benchmark.
SPARQL Query Processing System over Scalable Triple Data using SparkSQL Framework
MyungJoong Jeon, JinYoung Hong, YoungTack Park
Every year, RDFS data tends further toward scalability; hence, the manner of SPARQL processing needs to be changed for fast query. The query processing method of SPARQL has been studied using a scalable distributed processing framework. Current studies indicate that the query engine based on the scalable distributed processing framework i.e., Hadoop(MapReduce) is not suitable for real-time processing because of the repetitive tasks; in addition, it is difficult to construct a query engine based on an In-memory Distributed Query engine, because distributed structure on the low-level is required to be considered. In this paper, we proposed a method to construct a query engine for improving the speed of the query process with the mass triple data. The query engine processes the query of SPARQL using the SparkSQL, which is an In-memory based, distributed query processing framework. SparkSQL is a high-level distributed query engine that facilitates existing SQL statement. In order to process the SPARQL query, after generating the Algebra Tree using Jena, the Algebra Tree is required to be translated to Spark Algebra Tree for application in the Spark system, and construction of the system that generated the SparkSQL query. Furthermore, we proposed the design of triple property table based on DataFrame for more efficient query processing in the Spark system. Finally, we verified the validity through comparative evaluation with the query engine, which is the existing distributed processing framework.
Search
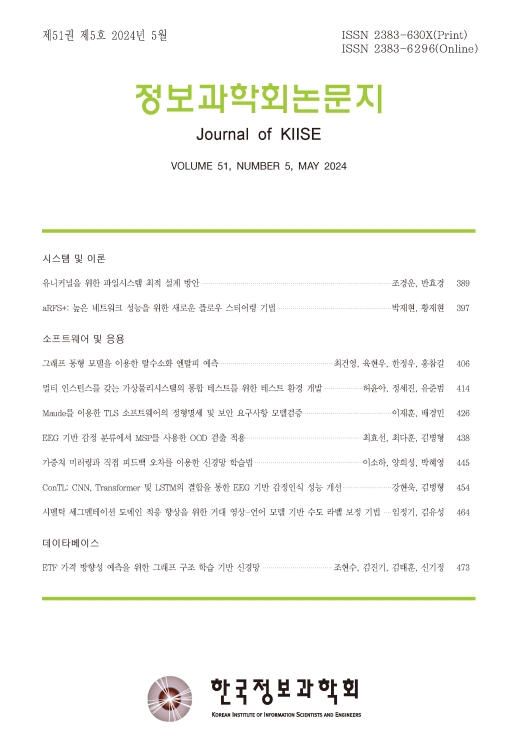
Journal of KIISE
- ISSN : 2383-630X(Print)
- ISSN : 2383-6296(Electronic)
- KCI Accredited Journal
Editorial Office
- Tel. +82-2-588-9240
- Fax. +82-2-521-1352
- E-mail. chwoo@kiise.or.kr