Digital Library[ Search Result ]
Data-Driven Computer-Aided Diagnosis of Ventricular Fibrillation Based on Ensemble Empirical Mode Decomposition of ECG
http://doi.org/10.5626/JOK.2020.47.4.387
In this paper, we propose a novel computer-aided diagnosis method to detect VF(ventricular fibrillation), one of the hazardous cardiac symptoms of arrhythmia by applying the EEMD(Ensemble Empirical Mode Decomposition) to the ECG signals. Using the EEMD to the ECG signals, it is shown that VF in the EMD region has a higher correlation with the IMFs (intrinsic mode functions) than the NSR (normal sinus rhythm) and other types of arrhythmia. To quantify this characteristic, we calculate the angle between the ECG signal and the specific IMFs, and classify the pathology by differentiating the angles. To verify the effectiveness of the proposed algorithm, we measured the accuracy of diagnosis using arrhythmia data from the PhysioNet database and confirm capacity of the proposed method.
Motor Imagery EEG Classification Method using EMD and FFT
David Lee, Hee-Jae Lee, Sang-Goog Lee
Electroencephalogram (EEG)-based brain-computer interfaces (BCI) can be used for a number of purposes in a variety of industries, such as to replace body parts like hands and feet or to improve user convenience. In this paper, we propose a method to decompose and extract motor imagery EEG signal using Empirical Mode Decomposition (EMD) and Fast Fourier Transforms (FFT). The EEG signal classification consists of the following three steps. First, during signal decomposition, the EMD is used to generate Intrinsic Mode Functions (IMFs) from the EEG signal. Then during feature extraction, the power spectral density (PSD) is used to identify the frequency band of the IMFs generated. The FFT is used to extract the features for motor imagery from an IMF that includes mu rhythm. Finally, during classification, the Support Vector Machine (SVM) is used to classify the features of the motor imagery EEG signal. 10-fold cross-validation was then used to estimate the generalization capability of the given classifier., and the results show that the proposed method has an accuracy of 84.50% which is higher than that of other methods.
Search
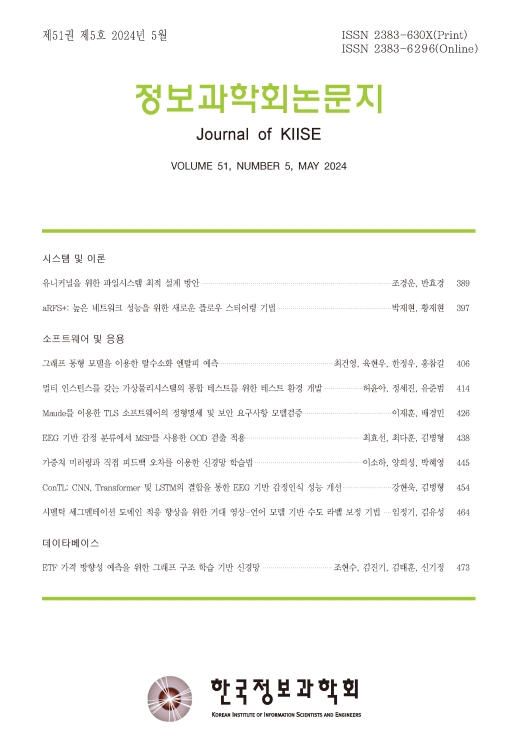
Journal of KIISE
- ISSN : 2383-630X(Print)
- ISSN : 2383-6296(Electronic)
- KCI Accredited Journal
Editorial Office
- Tel. +82-2-588-9240
- Fax. +82-2-521-1352
- E-mail. chwoo@kiise.or.kr