Digital Library[ Search Result ]
Motor Imagery Decoding with Residual Dense Network
Permana Deny, Sae Won Cheon, Kae Won Choi
http://doi.org/10.5626/JOK.2022.49.5.380
This article proposes a Residual Dense Network (RDN) framework for brain signals during motor imagery (MI) decoding. We designed a decoding framework including feature extraction and a decoding algorithm built on a deep neural network to perform feature learning and decision making. We analyzed the capability of the RDN to decode a public BCI dataset from BCI Competition IV Dataset 2A. Experiments were conducted to evaluate the capability in terms of the performance accuracy for a given dataset and showed that the RDN framework achieved a result of 0.8290, outperforming the previous study using the same dataset benchmark. In conclusion, the RDN provided a decoding framework in a practical brain-computer interface.
A Dual Filter-based Channel Selection for Classification of Motor Imagery EEG
David Lee, Hee Jae Lee, Snag-Hoon Park, Sang-Goog Lee
http://doi.org/10.5626/JOK.2017.44.9.887
Brain-computer interface (BCI) is a technology that controls computer and transmits intention by measuring and analyzing electroencephalogram (EEG) signals generated in multi-channel during mental work. At this time, optimal EEG channel selection is necessary not only for convenience and speed of BCI but also for improvement in accuracy. The optimal channel is obtained by removing duplicate(redundant) channels or noisy channels. This paper propose a dual filter-based channel selection method to select the optimal EEG channel. The proposed method first removes duplicate channels using Spearman"s rank correlation to eliminate redundancy between channels. Then, using F score, the relevance between channels and class labels is obtained, and only the top m channels are then selected. The proposed method can provide good classification accuracy by using features obtained from channels that are associated with class labels and have no duplicates. The proposed channel selection method greatly reduces the number of channels required while improving the average classification accuracy.
Filter-Bank Based Regularized Common Spatial Pattern for Classification of Motor Imagery EEG
Sang-Hoon Park, Ha-Young Kim, David Lee, Sang-Goog Lee
http://doi.org/10.5626/JOK.2017.44.6.587
Recently, motor imagery electroencephalogram(EEG) based Brain-Computer Interface (BCI) systems have received a significant amount of attention in various fields, including medicine and engineering. The Common Spatial Pattern(CSP) algorithm is the most commonly-used method to extract the features from motor imagery EEG. However, the CSP algorithm has limited applicability in Small-Sample Setting(SSS) situations because these situations rely on a covariance matrix. In addition, large differences in performance depend on the frequency bands that are being used. To address these problems, 4-40Hz band EEG signals are divided using nine filter-banks and Regularized CSP(R-CSP) is applied to individual frequency bands. Then, the Mutual Information-Based Individual Feature(MIBIF) algorithm is applied to the features of R-CSP for selecting discriminative features. Thereafter, selected features are used as inputs of the classifier Least Square Support Vector Machine (LS-SVM). The proposed method yielded a classification accuracy of 87.5%, 100%, 63.78%, 82.14%, and 86.11% in five subjects(“aa”, “al”, “av”, “aw”, and “ay”, respectively) for BCI competition III dataset Iva by using 18 channels in the vicinity of the motor area of the cerebral cortex. The proposed method improved the mean classification accuracy by 16.21%, 10.77% and 3.32% compared to the CSP, R-CSP and FBCSP, respectively The proposed method shows a particularly excellent performance in the SSS situation.
A Method of Feature Extraction on Motor Imagery EEG Using FLD and PCA Based on Sub-Band CSP
The brain-computer interface obtains a user"s electroencephalogram as a replacement communication unit for the disabled such that the user is able to control machines by simply thinking instead of using hands or feet. In this paper, we propose a feature extraction method based on a non-selected filter by SBCSP to classify motor imagery EEG. First, we divide frequencies (4~40 Hz) into 4-Hz units and apply CSP to each Unit. Second, we obtain the FLD score vector by combining FLD results. Finally, the FLD score vector is projected onto the optimal plane for classification using PCA. We use BCI Competition III dataset IVa, and Extracted features are used as input for LS-SVM. The classification accuracy of the proposed method was evaluated using 10×10 fold cross-validation. For subjects ‘aa’, ‘al’, ‘av’, ‘aw’, and ‘ay’, results were 85.29 ± 0.93%, 95.43 ± 0.57%, 72.57 ± 2.37%, 91.82 ± 1.38%, and 93.50 ± 0.69%, respectively.
Motor Imagery EEG Classification Method using EMD and FFT
David Lee, Hee-Jae Lee, Sang-Goog Lee
Electroencephalogram (EEG)-based brain-computer interfaces (BCI) can be used for a number of purposes in a variety of industries, such as to replace body parts like hands and feet or to improve user convenience. In this paper, we propose a method to decompose and extract motor imagery EEG signal using Empirical Mode Decomposition (EMD) and Fast Fourier Transforms (FFT). The EEG signal classification consists of the following three steps. First, during signal decomposition, the EMD is used to generate Intrinsic Mode Functions (IMFs) from the EEG signal. Then during feature extraction, the power spectral density (PSD) is used to identify the frequency band of the IMFs generated. The FFT is used to extract the features for motor imagery from an IMF that includes mu rhythm. Finally, during classification, the Support Vector Machine (SVM) is used to classify the features of the motor imagery EEG signal. 10-fold cross-validation was then used to estimate the generalization capability of the given classifier., and the results show that the proposed method has an accuracy of 84.50% which is higher than that of other methods.
Search
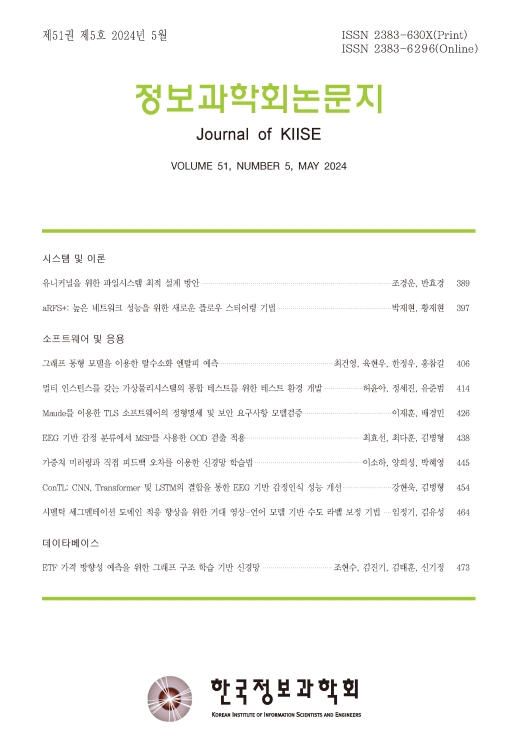
Journal of KIISE
- ISSN : 2383-630X(Print)
- ISSN : 2383-6296(Electronic)
- KCI Accredited Journal
Editorial Office
- Tel. +82-2-588-9240
- Fax. +82-2-521-1352
- E-mail. chwoo@kiise.or.kr